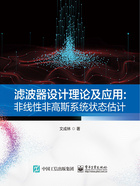
2.3 非线性高斯系统状态估计的无迹卡尔曼滤波器设计
对于基于系统非线性状态转移函数和观测函数切线逼近的一阶泰勒级数表示建立起来的扩展卡尔曼滤波器(EKF),随着系统模型或函数非线性程度的增加,系统状态估计精度会不断下降,甚至会出现滤波器发散的严重现象。为了提高滤波器对不同非线性函数的适应程度,Julier等人基于无迹变换的思想,于1970年提出了无迹卡尔曼滤波器(Unscented Kalman Filter,UKF)[1]。
无迹卡尔曼滤波器是无迹变换与标准卡尔曼滤波体系的结合,通过无迹变换使非线性系统方程适用于线性假设下的标准卡尔曼体系。UKF使用的是统计线性化技术,这一技术主要通过n个在先验分布中采集的点(称为sigma点)的线性回归来线性化随机变量的非线性函数,由于考虑的是随机变量的扩展,所以这种线性化技术比泰勒级数线性化技术(EKF所使用的技术)更准确。和EKF一样,UKF也主要分为预测和更新两个过程。
UKF的基本思想是卡尔曼滤波与无迹变换,它能有效地解决EKF估计精度低、稳定性差的问题,因为不用忽略高阶项,所以对于非线性分布统计量的计算精度高。
2.3.1 非线性系统描述
为简单起见,这里仅讨论下列情况下的非线性模型:

(2.3.1)

(2.3.2)
其中,w(k) 和v(k) 都是均值为零的白噪声序列。其统计特性如下:

(2.3.3)
在式(2.3.1)中

(2.3.4)
进一步,在式(2.3.2)中

(2.3.5)
另外,已知初始条件,即系统状态初始值x(0) 的统计特性如下:

(2.3.6)
2.3.2 无迹卡尔曼滤波器的建立
基于初始条件的统计特性及已获得的观测数据序列{y(1),y(2),⋯,y(k)} ,为了建立随时间递推的扩展卡尔曼滤波器,假设已获得系统状态x(k) 的估计值和相应的协方差矩阵:

(2.3.7)
下面给出UKF的推导过程。
1.构造以为中心的sigma点采样集合

(2.3.8)
其中,代表矩阵P(k|k) 经过数乘和开平方后的第i列。
相应的权重选择机制为

(2.3.9)
2.状态转移的时间更新
(1)基于式(2.3.7)产生的状态x(k) 的2n+1个sigma点,分别预测状态x(k+1) 的值。

(2.3.10)
(2)对由式(2.3.8)得到的状态x(k+1) 的2n+1个预测值进行加权融合,分别得到x(k+1) 的加权融合预测估计值和相应的估计误差方差矩阵。

(2.3.11)

(2.3.12)
3.测量更新
可以从两个方面开展对测量向量y(k+1) 的预测估计。
(1)利用由式(2.3.7)得到的关于状态x(k+1) 的2n+1 个预测估计值,对观测值y(k+1) 分别进行预测。
(2)基于式(2.3.11)得到状态x(k+1) 的预测值的估计误差协方差矩阵,采用式(2.3.7)的sigma点采样机制,得到2n+1 个状态x(k+1) 的预测值
。

(2.3.13)
对观测值y(k+1) 进行预测,得到

(2.3.14)
并进一步加权融合,得到

(2.3.15)
计算测量预测估计误差和测量预测估计误差方差矩阵:


(2.3.16)
进一步,计算状态预测误差与测量预测误差协方差矩阵:

(2.3.17)
4.求取卡尔曼滤波增益矩阵

(2.3.18)
设计无迹卡尔曼滤波器:

(2.3.19)
计算状态x(k+1) 估计值的误差和状态估计误差方差矩阵:

(2.3.20)

(2.3.21)
在滤波过程中,K表示滤波参数,调整它可以适当提升滤波估计的精度。对于n维状态向量,可得到2n+1 个采样点,可近似表示状态的高斯分布。而和
为权重系数,计算方式见式(2.3.9)。其中,L=α2(n+λ)−n。通常,α被设为一个较小的整数;λ是待给定的变量,通常设置为0或3−n;β表示分布变量,在高斯分布下一般设置为2,而在一维状态变量下通常取0。
UKF的采样方式是确定性采样,因而它仅需少量的样本点,对于n维状态向量系统,需要采集2n+1 个样本点。其计算量也并没有增加很多,在高维系统下性能更为可靠,但其面临与EKF同样的问题,即随着非线性增强而失效,同时并不适用于非高斯系统。
2.3.3 无迹卡尔曼滤波器的性能分析
1.UKF算法优势评述
实验分析表明,在正常运行的情况下,UKF可以实现接近泰勒展开的非线性二阶精度逼近,滤波性能优于EKF的一阶线性逼近,从而提高了对于非线性问题的处理能力。
2.UKF算法存在的问题与不足
UKF参数选择存在的缺陷常造成算法失效,主要表现为容易在三个矩阵中出现负定现象。分析如下。
(1)分析为什么会是负定的。
事实上,根据式(2.3.12),对于任意,有

(2.3.22)
其中,令,则

(2.3.23)
由于矩阵Q(k)≥0 是非负定的,因此,θTQ(k)θ≥0 。考虑到

(2.3.24)
所以有

(2.3.25)
若

(2.3.26)
就有

(2.3.27)
因此,正定与否依赖于
的选择,即若
,则
,否则,
将是负定的。当
时,将引发式(2.3.12)运行失效。
同理,对于矩阵也有类似的分析结果,它主要依赖于
的取值情况。
(2)分析是不是正定的。

(2.3.28)
如果是负定的,就不能正常求取增益矩阵:

(2.3.29)
从而造成算法在这一步失效。
(3)如果是正定的,
是负定的,那么在本次循环过程中,算法会在式(2.3.12)处失效。这是因为,欲使

(2.3.30)
就必须有

(2.3.31)
即

(2.3.32)
由于

(2.3.33)
事实上,对于任意,有

(2.3.34)

所以是正定的,因此要求
必须是正定的。
由上述分析可知,UKF算法所存在的问题,都是由式(2.3.9)中权重选取不当造成的,在实际仿真实验中,主要表现为和
出现负值。因此,选取合适的权重是UKF算法有效运行的关键。
(4)sigma采样描述了状态x(k) 的统计特性。UKF采样是基于状态x(k) 的估计误差协方差矩阵PU(k|k) 进行的,相比于EnKF无约束的随机采样来说,UKF采样是基于估计误差的统计特性得到的。但是在维度n较小时,采样点也很少,因此采样难以对状态x(k) 的统计特性具有代表性;当n较大时,得到的2n+1个采样点相对于n维空间来说是稀疏的,难以覆盖目标特性。