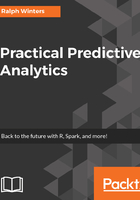
The Modeling Process
Today, we are at a juncture in which many different types of skill sets are needed to participate in predictive analytics projects. Once, this was the pure domain of statisticians, programmers, and business analysts. Now, the roles have expanded to include visualization experts, data storage experts, and other types of specialists. Yet, so many are unfamiliar with an understanding of how predictive analytics projects can be structured. This lack of structure can be inhibited by several factors. Often there is a lack of understanding of the critical parts of a business problem, and a model is developed much too early. Alternatively, a formal methodology may be put off to the future, in favor of a quick solution.
In this chapter, we will start by discussing the advantages of using structured analytics methodologies. Methodologies are a great way to demonstrate a value to the upper management, and groups or individuals advocating for a structured approach is one way to obtain buy-in from the management. If the project gains acceptance using a methodology, there is a strong possibility that future projects will have support.
We will cover the following points in this chapter:
- Advantages of a structured approach
- Analytic process methodologies
- An outline of the specific steps used within an analytics process methodology
- Short descriptions of specific analytic techniques, with code examples